Imagine one day in the future hiring a robot to come clean your home. To accomplish its prescribed task, the robot would need to navigate a potentially extremely cluttered environment, avoid or interact in a safe way with any people or pets moving in the space, and manage to clean and organize a variety of objects. This would likely involve interacting with other machines, and the robot would need to have a deep understanding of everything around it.
For Caltech artificial intelligence (AI) researcher Georgia Gkioxari, the challenges involved in such a task are endlessly exciting. Specifically, she is interested in using AI and machine learning to give machines (whether it be such a future robo-cleaner or something else) the ability to collect images as input and, from those, be able to understand things about the world around them—a field known as computer vision.
Meeting these challenges will be far from trivial, says Gkioxari, one of Caltech's newest assistant professors of computing and mathematical sciences and electrical engineering and a William H. Hurt Scholar. The human brain does an outstanding job of interpreting the sights captured by our eyes, allowing us to navigate, make decisions, and otherwise function in our complex world. "We should be thankful for all the previous generations and evolution that have led to us having this brain that allows us to process light into something meaningful. We don't have this for robots," she says. "Instead, we try to teach machines to learn from data, to use methods that digest large data sets—things like videos—to try to build meaningful representations that will allow them to act in it.
For her accomplishments in machine learning and computer vision, Gkioxari has recently been selected as a Google Research Scholar and was awarded an Amazon Research Award.
We recently spoke with Gkioxari about her work, the state of AI, and some of her favorite things about Caltech.
We have all witnessed a major shift in AI, where applications like ChatGPT are bringing their capabilities into the limelight. As someone who has been working in AI for more than a decade, how is it to be working in this field at this time?
A couple of years ago, we had a big result that led to a new state of the art in AI. I would say that we're still sort of in the wake of that; it hasn't really settled down. And it's an exciting era because we have unlocked techniques to learn well from data. This is what you see with ChatGPT, for example, techniques that learn really well from lots and lots and lots of data. We're still trying to understand how these models are working and finding applications of these models for other disciplines that have an abundance of data available.
We next need to figure out how to solve these types of problems in a way that requires much less data and computing. Most of the interesting problems are in cases where you don't have a lot of data to use for training. We humans are a great example of this. I can show you one object, and then you have learned it. You don't need billions of examples to learn it. If we manage to unlock that capability for machines, then I think we're talking about a revolution in our society.
What are some of the challenges that you are focusing on in your research right now?
There are many exciting directions that I'm equally thrilled to pursue. Right now, I'm really interested in bridging the gap between images and our four-dimensional world—being able, from an image, to understand the scene in 3D, meaning where objects are, how humans act within the scene, how they're moving, and how they interact with objects, etc.
I would love to unlock 3D perception. AI programs like ChatGPT are accomplishing great things with language. We are doing great things with images in the sense of generating images. You can tell a program, "Give me an image of a cat eating a hot dog," and you will get that. But it's the opposite that's important. I want to be able to give a program an image and have it tell me how far apart the objects are, what the shapes of the objects are, how the objects and agents are moving within the scene. These are the kinds of tasks that are very important for anything that has to do with embodiment for robotics applications. You don't care about generating an image like a cat eating a hot dog. What you care about is: Is this car coming toward me? Do I need to turn right or left?
How did you initially get interested in AI?
I come from Greece, and in my third year of undergrad studies there, I took a course that was called Pattern Recognition and Computer Vision. It was my initiation into this field of AI and computer vision. I was fascinated by the challenge of taking an image, which is just an array of colors stacked next to each other, and making it into something that's meaningful, like people's emotions or actions or objects. That is something that for you, because you have this human brain, is trivial. It's a seamless operation that you don't even think about. But how to operationalize that process into a computational system and model is very far from trivial, and that big challenge intrigued me.
After earning your PhD in computer vision at UC Berkeley, you worked at Facebook AI Research for six years. What made you want to switch to academia?
First of all, I spent six years in industry, which was plenty already. And I enjoyed every second of it. It was fantastic.
But my motto in life is, "You only live once." And I wanted to check out that gig that's called academia. I really enjoy working with students and mentoring them. The best part of my day is when I have research meetings with my students. I just love their youthful energy. It's very contagious.
I also wanted to see the purpose and the impact that AI can have, not just within an industry environment but in an academic environment. And that's why I picked Caltech—because of the importance that all the disciplines have and the interconnectedness between departments.
Have you been branching out to some of these other groups and divisions?
Absolutely, I have. The best way to do this is to go to all the Caltech events and chat with a bunch of people. There are a lot of opportunities for AI to have an impact in other scientific disciplines. So, the exercise that I'm going through right now is to pick the problems and the people that I enjoy collaborating with the most. Already I am working Hannah Druckenmiller, who is also a new faculty member in economics, where she wants to value ecosystem resources. Computer vision is a big part of that because you have visual data to figure out where the resources are, and then you also want to build machine learning models to figure out how to value them.
I'm also working very closely with Pietro Perona, who is a computer visual scientist but also works in neuroscience. And there are a lot of applications to ecology. We're working together on a project to count species in various parts of the US that have direct implications for our food chain. The first is a project to count all the salmon that go by in the rivers in Alaska. Right now there are people who stand on the shore and count the salmon. But we would like to have automated systems, so this is another area where you need a lot of good computer vision. Both of these projects, with Hannah and with Pietro, are supported by the Resnick Sustainability Institute at Caltech.
Soon-Jo Chung and I are also in the very initial stages of trying to bridge the gap between perception and robotics. We would like to have robots that are able to understand nighttime and daytime, and are able to navigate not just urban terrains but also nature, even navigating rivers.
Do you have any hobbies?
This is a question that we millennials hate. I will say that a lot of my creativity needs are being satisfied through my work, but I do like hiking a lot, and I also like food—not cooking it but eating it. This is what I love about LA. There are these amazing little eateries all over LA, often hidden away in strip malls, where you can find amazing Asian foods.
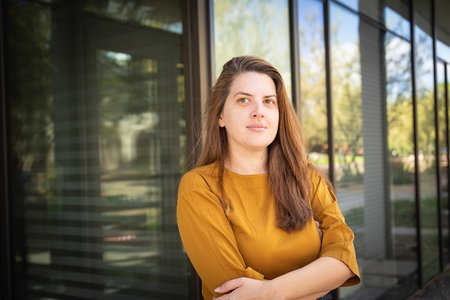
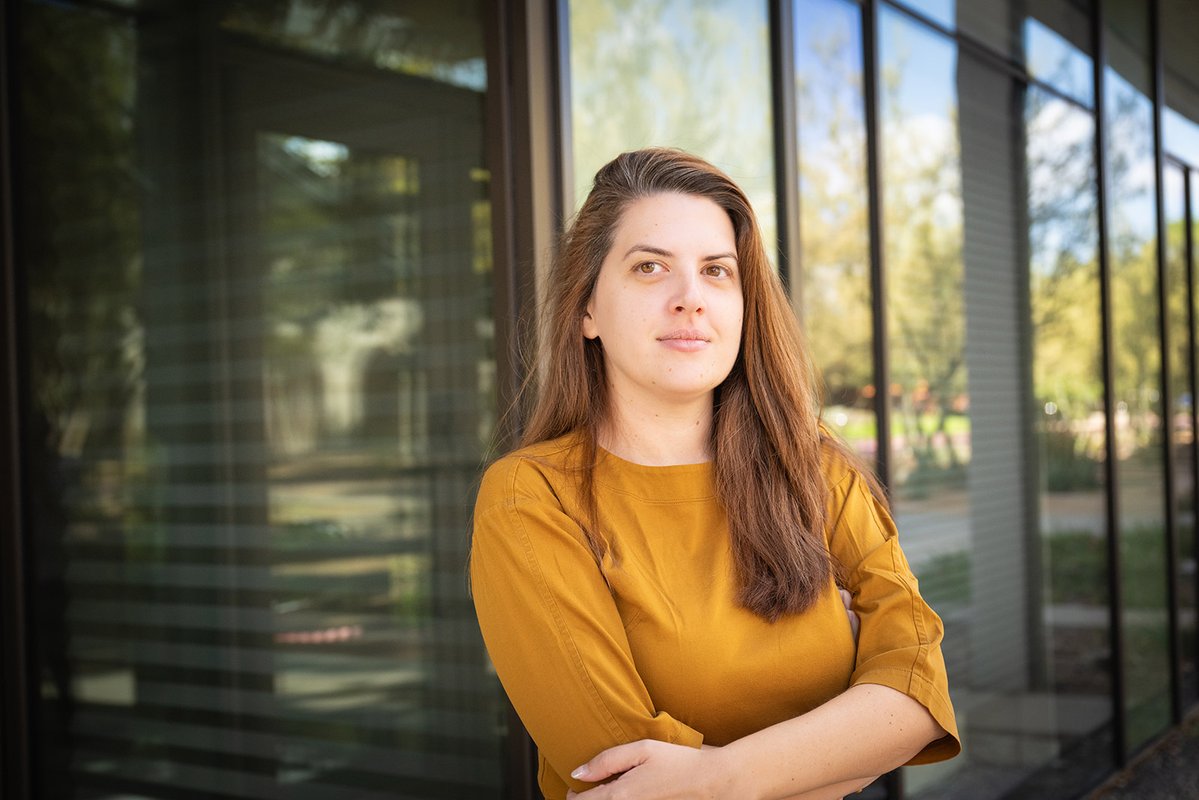