Joel A. Tropp, Professor of Applied and Computational Mathematics, will receive the Compressive Sampling Pioneer Award at this year’s International Society for Optics and Photonics Defense Security and Sensing conference (SPIE. DSS). He is one of the first researchers to contribute to the field of sparse approximation, which is also known as compressive sampling. At the conference he will give a presentation on sampling theorems for structured signals, based on his paper entitled “Living on the Edge.”
For over 40 years, researchers have studied methods for identifying a structured signal from linear measurements using convex optimization. The appropriate optimization problem depends on a priori information about the type of structure that is latent in the signal. Familiar examples include l1-norm minimization for recovering sparse signals and Schatten 1-norm minimization for recovering low-rank matrices, but there are many others. Extensive empirical work suggests that there is a phase transition in the number of measurements that are needed to recover a specific signal using convex optimization. Professor Tropp’s presentation will summarizes ideas from the last five years that allow researchers to compute the precise number of measurements required to identify a given type of structure. These results can be interpreted as sampling theorems for structured signals. He will also discuss recent research that validates the theoretical predictions in more applied settings. [Read the paper]
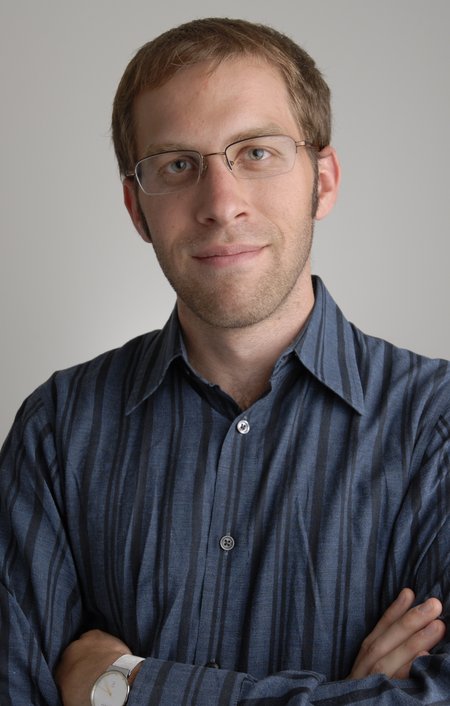
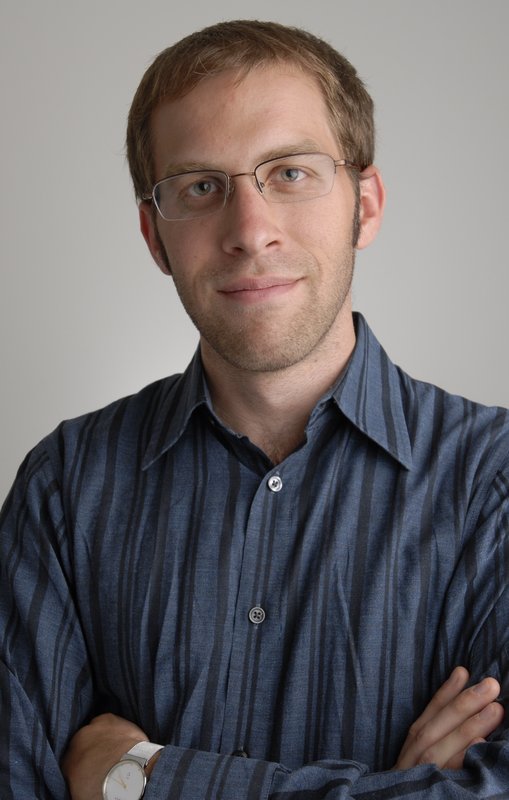